In 2050, there is a requirement to increase global food supply by 60 percent to feed nearly nine billion people. The growing population leads to continuous farming with limited arable land controverts with the 17 Sustainable Development Goals (SDGs) which have been focused on eliminating poverty and eradicating hunger and malnutrition by 2030 and 2025 respectively. Though intensive agriculture and industrial agriculture have led to an increase in food production and easing of food shortages, now bring disadvantages due to the utilisation of high input of fertilisers, pesticides and fresh water. How to feed the increasing population while decreasing the negative consequences on the environment and mitigating atmospheric changes is the biggest global challenge.
To safeguard food and ecological security, the sustaining of performing more of the same thing is commonly indicated as sustainable agriculture. Sustainability lies in three pillars economic, social and environmental performance. In agriculture, sustainable growth lies in four objectives namely 1) Ensure the production of an adequate food supply; 2) Alleviate poverty; 3) Achieve better health and nutrition for a growing population; and 4) Conserve natural resources. These objectives are highly relevant to the sustainable pillars, and they are aligned properly with the SDGs.
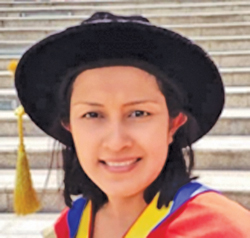
Dr. Vilani Sachithra
To meet sustainable objectives and face the global food security challenges ahead, wider application of existing technologies and utilisation of advanced technological tools and techniques soon would be the straightforward strategies. The recent application of the technologies of Artificial Intelligence (AI) models supports to provision of solutions to problems in the agricultural domain. These technologies are used to reduce the cost as well as increase effectiveness and efficiency. With the development of AI models, there is a growing need to use them to achieve sustainable agriculture.
While there is a plethora of information about how AI may provide value to agriculture, research on how AI is used to achieve sustainable objectives is evolving. Thus, the main purposes of this systematic review are too; 1) develop a more complete understanding of the enabling AI technologies currently applied in the agriculture sector, 2) explore a variety of AI technology initiatives to achieve sustainability growth objectives, and 3) analyse how agriculture firms/farms improve sustainable growth through technologies which are already underway and new technologies are being developed.
Method
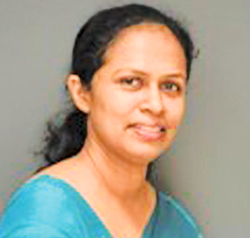
Dr. Sashikala Subhashini
To carry out this review, we set up three stages namely, planning; implementing and reporting. In the planning stage, the key terms that could be considered related to the study namely, agriculture, farming, protected agriculture, smart farming, Artificial Intelligence (AI), Deep Learning (DL), Machine Learning (ML), agricultural robots and robotics. In the implementation phase, 1421 articles were selected with the search tool. During this process, the list of documents was consequently sorted to eliminate the duplicate articles and 115 articles deemed eligible as a sample for this study. In the reporting stage, each of the articles retrieved was analysed according to the AI component such as DL, ML, ANN and robotics and agriculture activities namely harvesting, plant ecophenotyping, grading system, weed and crop classification, disease detection and monitoring and soil management.
AI methods used in agriculture
In our analysis, we found that the main AI approaches used in agriculture are Artificial neural networks (ANN): Deep learning (DL):Fuzzy Logic, Support Vector Machine (SVM), Random Forest, K-nearest and Robotics. Robots are used in agriculture for weeding applications, visual detection and harvesting. DL apply to classify the fruit image, identify seeds and pests, monitor nitrogen content in soil and leaf, detect irrigation and plants’ water stress level, assess erosion of water, detect usage of herbicide, defects on food and damage of crop hail and monitor greenhouse. ANN applies to take various soil and environmental parameters and predict hourly requirements of soil moisture content.
Agriculture functions and AI
The most common applications of AI are predicting, harvesting and advanced care of crops. Of the total 115 studies, 36 (40 percent) articles reported that the most common applications of AI for agriculture are prediction models for total agricultural output value, waste minimisation, weather index, energy optimisation, and demand and consumer preference. ANN and DL techniques are used by 95 percent of the reviewed papers. These preference predictions provide wider chances to develop food product designs in future. Here, agriculture sustainability is ensuring the production of an adequate food supply.
Harvesting is a challenging task in agriculture because harvesters strongly correlate to crop detection, quality cuts, damage, picking and packaging. Robotic technique (robot arm) has been identified as an effective tool for assistance in the agriculture industry to harvest fruit/vegetables. Harvesting robot concentrations on five key areas; identifying targets under complex background; separating soft crop; level of consuming energy to harvest, harvesting tools suitability and conformation design to fit with unshaped work fields. Development of timely, efficient and careful robotic harvesting solutions leads to the completion of the harvesting process. This generates high-quality yields at minimum time-consuming and at minimum un-recuperative damages. Particularly, there have been significant developments of AI towards the sustainability agriculture objective of ensuring production of an adequate food supply.
Advanced care of crops is another agricultural function where AI is mostly applied (26 articles; 29 percent). AI technologies, such as DL, ANN, robotics and ML, provide the means to automate disease detection, measure plants, monitor plant growth status and apply fertiliser. The robotic disease detection systems were commonly designed in a whole inclusive pattern to identify the results of infection and these results could be utilised to detect precise diseases and apply fertilisers appropriately. It is important to recognise the AI applications that favour both disease management and provide sufficient, safe, and nutritious food to the global population. Particularly, there have been significant developments in AI towards the sustainability agriculture objective of achieving better health and nutrition for a growing population.
Womb of agriculture is soil and soil management therefore serves as a primal concern in agricultural resource management; ANN, DL, ML and robotic techniques are widely used by the reviewed papers. Ground robots and UAVs are more precisely used to collect soil and water samples and land preparation/sowing. ANN, DL and ML techniques are used to compute the normalised soil moisture index to estimate the soil moisture content and develop a model to assess the agricultural land for cultivation. Estimating evapotranspiration, stream flows and real-time management of reservoir release by using ML algorithms are highlighted in the review as irrigation management.
Considerations
In summary, Figure 2 tabulates the contextual link of usage of AI techniques that are supposed to address sustainable agriculture. The number of studies that address sustainable agriculture is increasing; however, less attention has been devoted to investigating the sustainability aspect of agriculture about AI. Besides research projects that have been performed, very few have developed into the commercial world (Kiwi fruit; Tomato; Cotton; Apple; Rice).
Unsustainable agricultural production practices such as food wastage and production shocks can be minimised if the sector uses AI to get accurate predictions. Development of timely, efficient and careful robotic harvesting solutions leads to obtaining desired quality harvest in short period and more interestedly at a minimum unrecoverable loss. AI applications in natural resources management (such as water, soil, and land) are presently at an unsatisfactory level. The final consumer aspect in the agriculture supply chain needs to be devoured with extensive attention to achieve one of the key agriculture sustainability objectives; alleviating the poverty of farmers. Moreover, developing a specific prediction model for market demand and preference for agriculture products is insufficient. Due to the low repeatability and difficulties in corresponding, AI implementation in the agriculture sector becomes the main challenge.
The usage of AI and image processing techniques has become more common to improve the sustainable agriculture. When considering the DL models, these models suffer from task dependence since all the models use general word embedding vectors. To overcome this problem attention-based DL models can be developed. Future studies should intend to address the gaps identified in this systematic review such as under-utilised commercial crop appraisal, data capturing conditions, natural resource standards, functional areas and geographical locations.
Figure 1: AI techniques in addressing sustainable agriculture
This is an extract from the journal article published; by V. Sachithra and L.D.C.S. Subhashini (2023). How artificial intelligence used to achieve agriculture sustainability: Systematic review, Artificial Intelligence in Agriculture, Vol. 8, pp. 46-59. https://doi.org/10.1016/j.aiia.2023.04.002
By:
Dr. Vilani Sachithra
Senior Lecturer, Department of Commerce, Faculty of Management Studies and Commerce, University of Sri Jayewardenepura, Sri Lanka
Dr. Sashikala Subhashini
Senior Lecturer, Department of Information Technology, Faculty of Management Studies and Commerce, University of Sri Jayewardenepura, Sri Lanka